
A Comprehensive Guide to Predictive Maintenance in Manufacturing | 2023
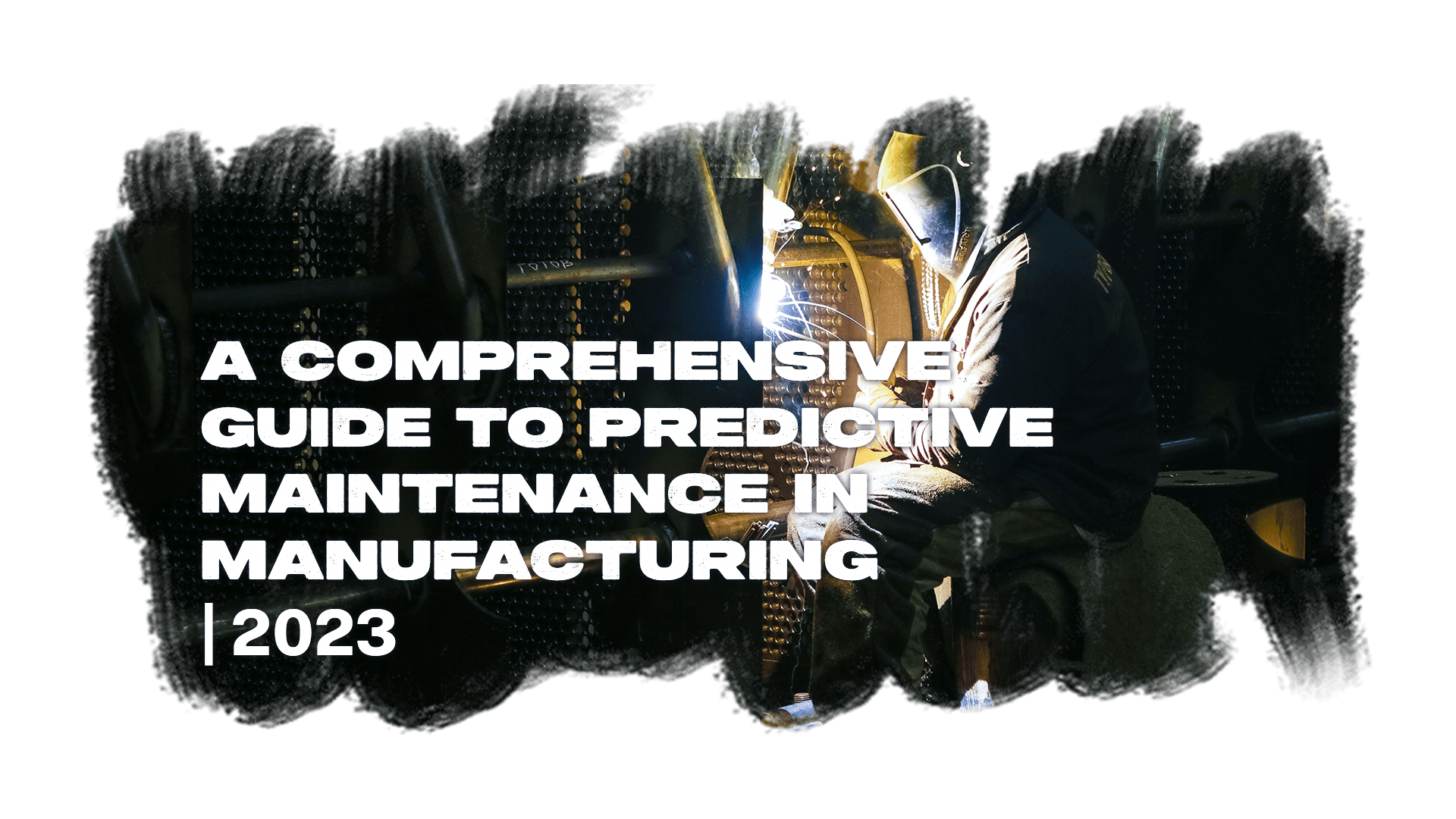
Effective maintenance requires a well-rounded approach. Manufacturing companies use planned, reactive, and proactive systems to prevent breakdowns and address problems as soon as they arise.
Table of Contents
1. Fundamentals of Predictive Maintenance
2. Predictive Maintenance in Manufacturing – Techniques
3. The Steps of Predictive Maintenance
4. Data in Predictive Maintenance in Manufacturing
5. Predictive Maintenance Challenges
6. Predictive Maintenance Best Practices
7. Predictive Analytics Techniques
8. Predictive Analysis Applications
9. Predictive Maintenance Implementation Process
10. Future Trends & Emerging Technologies
With the rise of machine learning (ML), big data, and its numerous applications in manufacturing settings, predictive maintenance is quickly becoming a critical tool.
Predictive maintenance allows for a proactive approach driven and backed by real-time data.
Using advanced monitoring techniques that analyze the condition of a piece of equipment, predictive maintenance can anticipate the optimal time for performing maintenance tasks, meaning right before a piece of equipment breaks down or before a failure occurs.
In return, organizations get a slew of benefits, including:
- Extending asset lifetime
- Reducing the cost of replacement parts
- Fewer equipment failures, meaning less unplanned downtime
- Reduced Mean Time to Repair (MTTR)
- Precise asset data
- Verification of repair efficiency
- Improved workplace safety
- Increased Return on Investment (ROI)
Studies show that unplanned downtime costs organizations around $50 billion annually and can reduce production capacity by as much as 20%. It’s easy to see the benefit of having a system that can cut back on those numbers.
Predictive maintenance can provide a long list of benefits, like 5-10% savings on material costs, between 10%-20% reduced maintenance planning time, and a 5%-10% reduction in overall maintenance costs.
While implementing predictive maintenance in manufacturing can cost a bit more upfront, the long-term cost savings and benefits are significant enough to make it worth the expense for most companies.
In this article, we will break down the strategies involved in predictive maintenance, explain how it works, and how to get started.
Fundamentals of Predictive Maintenance
Predictive maintenance, as it looks today, is relatively new, made possible in part by the rise of technology in manufacturing. But the practice has been around since the early 21st century, starting as more of an "offline" course with periodic measuring and comparison.
Today, predictive maintenance involves constant, real-time monitoring provided by IoT sensor devices and maintenance software that tracks the condition and performance of a piece of equipment.
This allows for statistical analysis of that data and the ability to predict when a breakdown is likely to occur – before it happens. Data eliminates some guesswork from the maintenance system and helps organizations plan maintenance activities more efficiently.
Predictive Maintenance in Manufacturing – Techniques
Vibration Analysis – Machinery that experiences vibration due to rotating parts can suffer from wear and tear. Sensors that monitor the effects of that vibration, such as loosening, can pinpoint instances where machinery is becoming out of balance or misaligned – a condition that can quickly lead to breakdowns and failure.
Acoustic Analysis (Sonic and Ultrasonic) – Measuring the acoustic sound produced by bearings during operation can help predict when lubrication is required to prevent mechanical failure. Ultrasonic sensors, however, can detect subtler sounds emitted by electrical or mechanical equipment related to friction or stress.
Infrared Analysis – Infrared sensors track the temperature of certain assets and can identify problems related to stress, airflow, and cooling.
The Steps of Predictive Maintenance
- Establish Baseline Measurements – Establish a baseline before installing sensors. This allows easier detection of measurements that fall outside of peak-condition performance.
- Implement IoT Devices – Install the most relevant and useful sensors on the equipment (vibrational, acoustic, or infrared).
- Connect IoT Devices to Maintenance Software – A CMMS (computerized maintenance management system) allows data collection from the IoT sensors and analysis of that data to predict future failures or breakdowns.
- Schedule Maintenance Based on Pre-Set Triggers – When a measurement falls outside of the acceptable conditions for operation, it triggers the need for scheduled maintenance.
Adding Up the Savings
According to the US Department of Energy, predictive maintenance can "eliminate catastrophic equipment failures." The body found that organizations implementing a predictive maintenance program see a 70%-75% elimination of breakdowns.
The same study found a 10X increase in ROI, a 25%-30% reduction in maintenance costs, and a 35%-45% reduction in downtime.
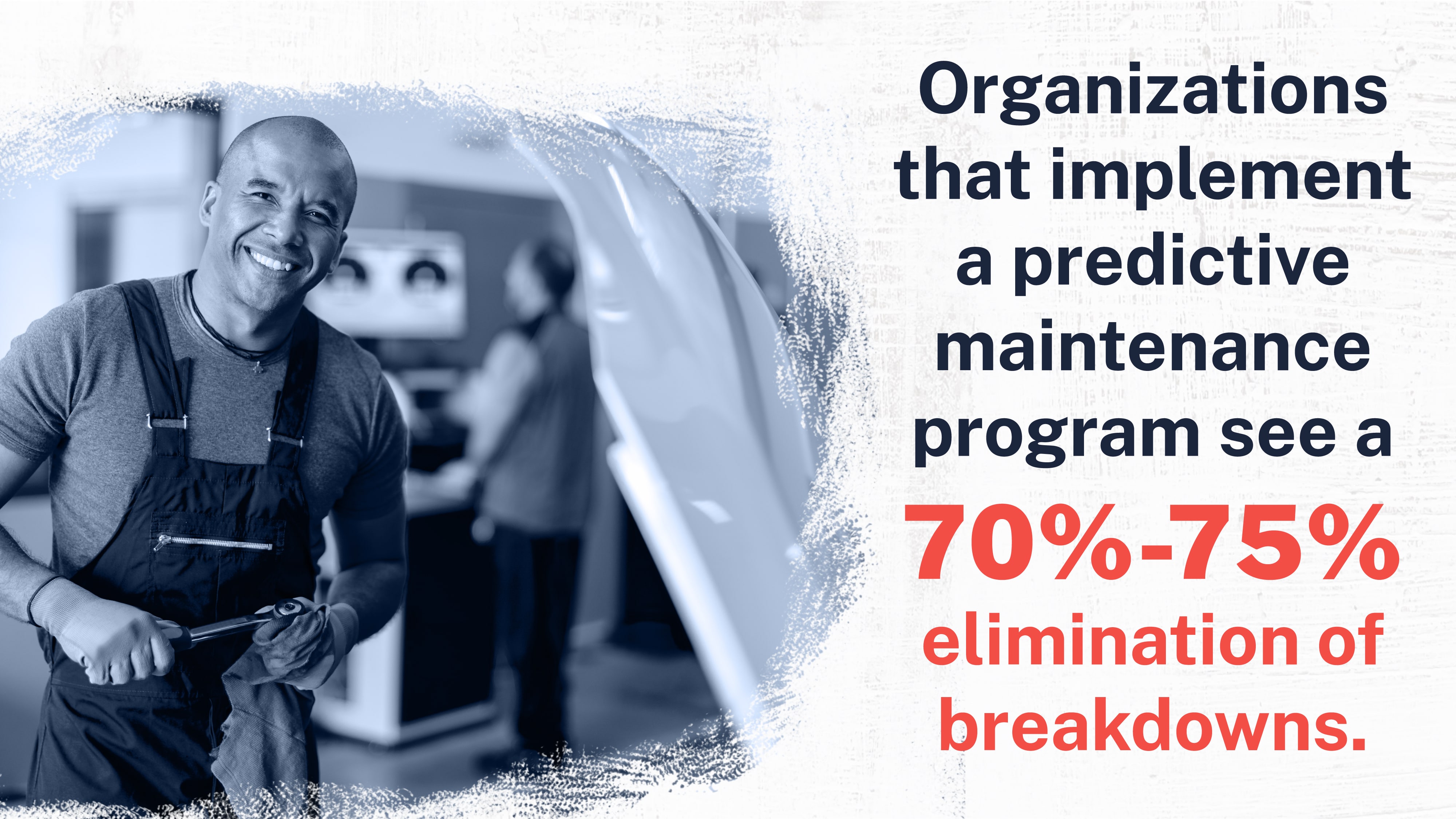
Data in Predictive Maintenance in Manufacturing
At the crux of predictive maintenance in manufacturing is data. The strategy behind the system is reliant on real-time data to predict when equipment will fail. The accuracy of the predictions is critical to helping organizations save time and money when scheduling maintenance activities.
The IoT and smart sensors have enabled manufacturers to collect more data than ever. But for that data to be helpful, organizations must analyze it effectively.
Statistical analysis involves looking for patterns and trends in a collection of data. Manufacturers can use two main methods to predict the need for maintenance. The most appropriate for a particular scenario is essential in analyzing biomedical data.
Parametric Analysis
Parametric statistics use a set of fixed parameters, or assumptions, that relate to a particular dataset. In other words, we know certain things about the population from which the data was drawn to be true or "normal." Given those "normal" parameters, we can create a probability model that relies on what we know to be true about the population – or in this case, a piece of machinery or equipment.
Non-Parametric Analysis
Non-parametric statistics are those that are not dependent on the population from which the dataset was obtained. That means there are no fixed parameters and no fixed distribution method. Non-parametric analysis requires no assumptions, which means this method can be applied easily, and in more applications.
Machine Learning is Running Big Data
Gone are the days when statistical analysis was performed manually by a group of pencil pushers. Big data has made that method ineffective and almost obsolete. Instead, organizations are turning to machine learning technology to collect, organize, and analyze large datasets.
Machine learning uses models built with a specific purpose. Through this method, manufacturing companies can make better use of the available data and use it to predict when a piece of machinery is likely to fail.
Predictive Maintenance Challenges
Predictive maintenance in manufacturing is an evolving field. Because of its uses and benefits, it has become a front-line defense against mechanical breakdowns – and a buffer against the cost of lost productivity related to downtime and maintenance activities.
Any new system is going to face challenges. Trial and error have already revealed a few lessons that manufacturing companies can use to their advantage.
Here is a list of some of the most common challenges of implementing predictive analysis.
Data Collection and Analysis
Predictive analysis relies on big data to work correctly and requires the practical study of that data. Collecting the correct data is the first step. Understanding how to analyze and use that data is the second. Both components must be in place and working together for predictive maintenance to be effective.
Lack of Skilled Workers
A new and growing field will require skilled workers to implement and operate it. A lack of workers with the skills, training, and knowledge to use and apply these systems can hamper their usefulness.
Integration
Part of the challenge of implementing new and advanced technologies into an organization is integrating them with existing systems. This is often an overwhelming task for manufacturing companies because of their size and scope. Integration can be time-consuming and costly to carry out.
High Implementation Costs
Speaking of costly, predictive maintenance comes with a significant upfront investment. IoT sensors, CMMS software, and the skilled workers to implement these things can be pricey. But the long-term benefits often outweigh the investment – if organizations can put forth the effort to get it right.
Predictive Maintenance Best Practices
Pinpoint Losses for Key Machines
The high cost of implementing predictive maintenance often requires a slow rollout. Pinpointing the most crucial applications can help mitigate the cost. Focus on critical pieces of machinery, or those suffering significant losses because of breakdown or failure, first.
Collect Data
Start with gathering the right data using the best methods. IoT sensors can collect data through different methods. Identifying which method is best suited for each type of machinery is key.
Analyze Data
Once the sensors are in action and collecting data, it’s time to put it to work. That means analyzing it using the most effective method. Data can help predict when a piece of equipment will fail, but it also has other uses. For example, data can calculate the true cost of downtime and slowdowns, point to problems with suppliers and materials, and much more.
Identify Trends
Statistical and data analysis helps to identify trends and patterns that, in turn, can help predict future outcomes. When A plus B tends to equal C more often than not, it’s reasonable to assume that the result will be similar.
Act
Predictive maintenance works by allowing organizations to streamline their maintenance processes for the best outcome. Using real-time data to predict future outcomes is great, but only effective if that information is implemented.
Predictive Analytics Techniques
We’ve covered the basics of predictive analytics in manufacturing. Now it’s time to get into some specifics. There are several different techniques that predictive analysis employs, and each is targeted to an individual problem. Here are the techniques of predictive statistical analysis:
Classification – Classification techniques are helpful for determining the category of a potential event. For example, determining customer actions or customer churn.
Regression – Regression techniques are used to predict a numerical value. An example would be the number of sales made per month.
Clustering – Clustering models group similar data points, making it easier to recognize direct correlations.
Anomaly Detection – Anomaly detection techniques help recognize data points that are unusual or unexpected.
Predictive Analysis Applications
Predictive maintenance is one potential application of predictive analysis. But organizations of all types are taking advantage of greater access to real-time data to improve their performance and make better decisions.
Here are some examples of predictive analytics in action:
Fraud Detection – Predictive analysis can help pinpoint and identify fraudulent transactions by identifying patterns in behavior.
Risk Assessment – Businesses that face risks can use predictive analysis to weigh the potential outcome of a decision. An example would be assessing the risk of an event, such as a customer defaulting on a loan.
Customer Churn Prediction – Customer acquisition is expensive, so organizations must take steps to prevent customers from jumping ship. Predictive analysis can help identify customers who are likely to leave a business.
Asset Maintenance – The most useful application of predictive analytics for manufacturing companies is asset maintenance. Predicting when an asset is expected to fail allows maintenance scheduling before the failure occurs.
Predictive Maintenance Implementation Process
Implementing predictive maintenance in manufacturing is a multi-step process. Getting it right requires careful planning and a well-thought-out approach. Instead of jumping in headfirst, a strategic and slow rollout is usually easier to manage and allows for some trial and error on a smaller scale.
Follow these steps for a successful launch.
1. Identify
Start by identifying the most helpful application for a predictive maintenance program – the piece of machinery high up in a chain that other machines rely on or the asset that costs the organization the most when it goes down. Start with the applications that will most affect cost and productivity.
2. Collect
Data comes from several different types of sensors, such as vibration sensors and temperature gauges. Choose the data collection method that makes the most sense regarding the type of machinery in question. And remember that the more data you collect, the more accurate the predictive maintenance model will be.
3. Analyze
Use statistical models and machine learning to organize and analyze the data collected. Look for patterns and trends to identify potential future outcomes and predict when a piece of machinery will fail.
4. Act
Use the information obtained to implement a maintenance solution. Gather data, analyze that data to predict when a failure will occur, and then take action to prevent that failure from occurring.
Future Trends & Emerging Technologies
Predictive maintenance in manufacturing is one of the fastest-growing and most valuable applications of smart technology for organizations. But it’s still a relative infant – as is much of the advancing technology being created and implemented today. Progress moves quickly, and many manufacturers are stumbling to keep up.
Machine Learning – Revolutionizing Manufacturing
One of the most significant learning curves for organizations is using machine learning. ML has rapidly become intertwined with the fabric of manufacturing in 2023, and the future will only see its uses increase.
Machine learning has incredible potential to help manufacturers streamline operations, optimize production processes, and improve quality control. And as we’ve just discussed, ML can predict future equipment failures accurately, allowing for more effective and efficient scheduling of maintenance activities.
If it doesn’t sound like it from that list of applications, ML has the potential to revolutionize manufacturing – from corner to corner. The industry relies on building high-quality and affordable consumer products, and ML helps facilitate that.
The IoT – Connecting Manufacturing to the Future
The IoT, or the Internet of Things, is rapidly transforming manufacturing facilities. Connecting individual parts of an operation, incorporating data and analytics, and improving the tools and technology that manufacturers use are revolutionizing the industry for the better.
The IoT and predictive analytics work together to give organizations access to data they otherwise would never have access to. That information is invaluable to manufacturers. It can help improve decision-making, optimize operations, and prevent problems before they occur. These applications and systems are only going to get better from here.
Final Thoughts
PdM, or predictive maintenance, is a powerful tool that can help organizations improve and strive for that ideal of continuous improvement. Predictive maintenance in manufacturing helps companies avoid costly downtime and equipment failures and improve the lifetime of assets. Eliminating these expenditures and increasing productivity allows organizations to reallocate those funds elsewhere.
The data is out there. Now that tools and systems are available via the IoT and ML, organizations can access that data and put it to use. The outcome is obvious and will only grow as more organizations learn how to implement PdM systems effectively. It might cost some time and money upfront, but the results show that the benefits extend to both the company and its customers.
The tip of the iceberg is reliably available and affordable products of a higher quality for the customers and a more significant ROI for the manufacturing companies. Only the future will tell how far this type of technology will go.
Related Posts
View All PostsMaximizing Efficiency: A Guide to Total Productive Maintenance
Maintenance is an essential requirement for any manufacturing company. Whenever machinery and equipment enter an equation, there will be a likelihood of that machinery...
Continue ReadingImpact of AI in Manufacturing: Benefits, Challenges & Use Cases
Artificial Intelligence has become a hot topic in society today. What once was a term relegated to science fiction novels is now commonplace among people from all walks of...
Continue ReadingAPI: Making Your Software Work For You
What is an API? For those of us outside the world of programming, the term “API” may seem like an arbitrary acronym that your IT department likes to throw around. However,...
Continue Reading